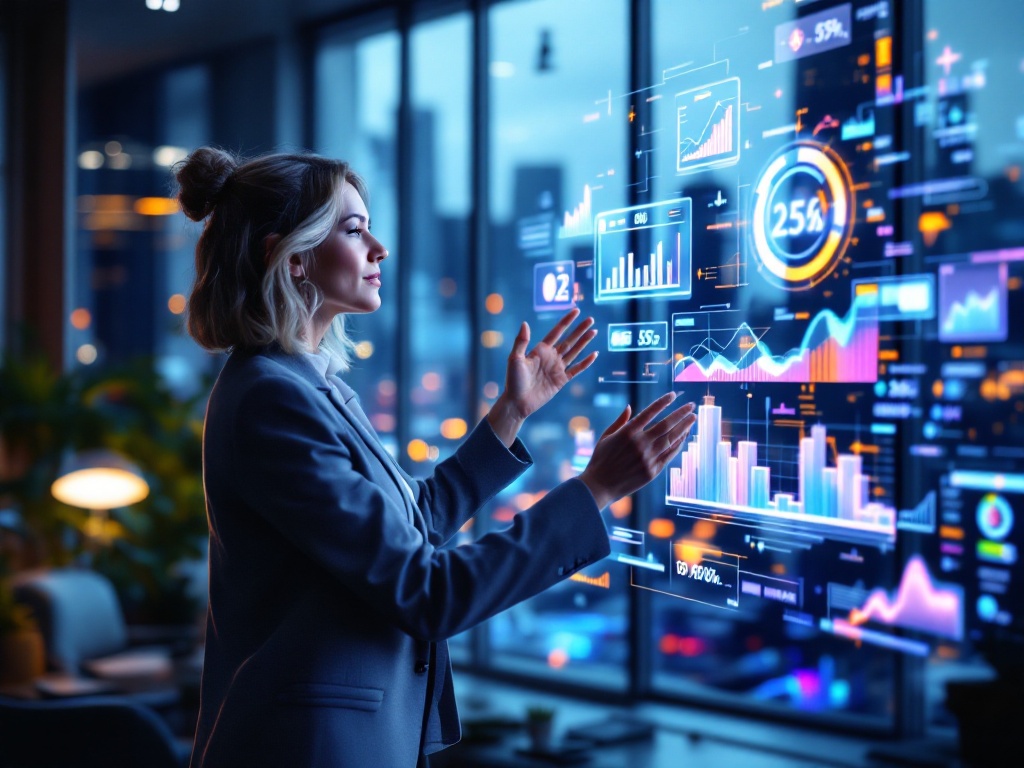
Introduction to Financial Forecasting
Financial forecasting is a critical process for entrepreneurs aiming to steer their businesses toward growth and success. It involves the estimation of future financial outcomes based on historical data, economic trends, and current market conditions. By utilizing various methods to predict revenue, expenses, and cash flow, entrepreneurs can make informed decisions that sustain their business operations and strategic initiatives.
The advent of AI forecasting and machine learning technologies has profoundly transformed the landscape of financial forecasting. Traditional methods often relied heavily on instinct and experience, supported by basic statistical analysis. Today, advanced algorithms can analyze vast datasets more efficiently and accurately than ever before. Entrepreneurs can now leverage these technologies to gain insights that not only enhance precision but also reduce the time spent on manual forecasting processes.
Machine learning, specifically, empowers systems to learn from data patterns and improve over time. This adaptability is crucial for financial forecasting since market variables frequently shift due to economic fluctuations, changes in consumer behavior, or unexpected global events. Such algorithms can identify trends and anomalies that might elude human analysts, making them invaluable tools in an entrepreneur’s arsenal.
Moreover, the integration of financial technology (FinTech) further complements the capabilities of AI and machine learning in this domain. Platforms equipped with these technologies can automate the entire forecasting cycle, from data collection to analysis and reporting. This not only streamlines operations but also enhances accuracy, allowing business owners to focus on strategic initiatives rather than spending excessive time on the complexities of forecasting.
Looking ahead, the significance of AI and machine learning in financial forecasting continues to grow. As these technologies advance, they promise even greater enhancements in precision, scalability, and usability for businesses of all sizes. In the upcoming section, we will explore the historical overview of financial forecasting methods, laying the groundwork to understand how these revolutionary changes came to be and what they mean for future financial practices.
Historical Overview of Financial Forecasting Methods
Financial forecasting has a rich history that reflects the evolution of economic theory and technology. In its earliest form, forecasting relied on basic human intuition and qualitative assessments. Historically, entrepreneurs often based their financial predictions on anecdotal evidence, personal experiences, and simple judgment calls. This rudimentary approach was limited by data availability and the ability to analyze it effectively, leading to predictions that could be quite inaccurate.
As commerce evolved, so did the methods of forecasting. By the mid-20th century, entrepreneurs began to embrace formalized statistical techniques, such as linear regression and time-series analysis. These approaches allowed for a more systematic collection of data, providing financial analysts with tools to quantify relationships between variables. For instance, businesses started to track sales over time, measuring them against relevant economic indicators. However, the requirement for consistent data and the inherent challenges of identifying underlying patterns meant that such methods still bore limitations in adaptability and accuracy.
With the advancement of computing technology in the latter half of the century, financial forecasting gained a new dimension. The introduction of complex algorithms facilitated more sophisticated modeling techniques, allowing for the incorporation of multiple variables into forecasts. During this era, the rise of econometrics – the application of statistical methods to economic data – paved the way for increasingly complex forecasting techniques. Still, these methods remained largely static, relying on predefined models that struggled to adapt to rapidly changing market conditions.
The dawn of the digital age in the late 20th century and early 21st century brought a substantial transformation to financial forecasting. The rise of databases and data warehousing allowed for the aggregation of vast amounts of information from various sources. This period marked a significant turning point, as businesses gained access to real-time data, enabling them to refine their forecasting techniques. However, the challenge of processing and analyzing this data with traditional methodologies persisted, leading to increased demand for innovative solutions.
The introduction of AI forecasting and machine learning fundamentally redefined financial forecasting once again. These technologies not only automate data analysis but also enable systems to learn and improve from ongoing data inputs. For instance, machine learning algorithms can identify complex patterns and correlations in data that traditional methods might overlook, such as sudden shifts in consumer behavior or market disruptions. This ability to adapt to new information makes machine learning particularly robust against volatility in the financial market.
Moreover, financial technology (FinTech) enhances the integration of AI and machine learning, pushing the boundaries of data utilization. FinTech platforms now incorporate predictive analytics, adapting dynamically to changing market conditions in ways that were previously unattainable. Through automation, these solutions streamline forecasting cycles, allowing entrepreneurs to allocate their resources more effectively to strategic decision-making.
The continuous advancements in AI and machine learning have made it increasingly clear that the future of financial forecasting is not just about enhancing accuracy; it is about enabling agility in decision-making processes. As entrepreneurs become more familiar with these technologies, they can harness their capabilities to anticipate market shifts, optimize their operations, and ultimately drive sustainable growth.
As we look ahead to the next section, we will delve deeper into the impact of technology on financial forecasting, examining how these innovative advancements are reshaping not only the accuracy of forecasts but also the overall strategic landscape for entrepreneurs.
The Impact of Technology on Financial Forecasting
The convergence of technology and financial forecasting has catalyzed an unprecedented shift in how entrepreneurs navigate the complexities of market predictions. With the advent of financial technology, the landscape of forecasting has become more sophisticated and efficient than ever before. Traditional forecasting methods, which often relied heavily on historical data and static models, are increasingly being supplemented or replaced by AI forecasting and machine learning techniques. These innovations allow businesses to harness the power of vast data sets and derive insights that were previously unattainable.
The ability of AI and machine learning to analyze and interpret data goes beyond mere number crunching; they are designed to identify patterns, detect anomalies, and even predict future trends based on real-time data inputs. This dynamic capability is particularly essential for entrepreneurs operating in fast-paced sectors where market conditions can change significantly within short timeframes. For example, machine learning algorithms can analyze consumer buying behavior, social media trends, and economic indicators simultaneously, thereby providing a holistic view that supports more informed decision-making.
One of the most significant impacts of technology on financial forecasting is the shift towards predictive analytics. By leveraging advanced algorithms, entrepreneurs can forecast financial performance with improved accuracy. Predictive models can help businesses analyze prospective sales, cash flow, and inventory needs while taking into account seasonal factors and economic fluctuations. As a result, entrepreneurs are empowered to make strategic decisions grounded in data-driven insights, fostering both agility and innovation.
Additionally, the integration of AI in financial forecasting leads to the automation of routine tasks, such as data collection and processing. This automation reduces the time spent on manual analysis, allowing entrepreneurs to focus on strategic initiatives and growth opportunities. Financial technology platforms provide user-friendly interfaces that enable even those without substantial technical expertise to access powerful forecasting tools, democratizing the ability to predict financial outcomes effectively.
Furthermore, the scalability offered by these technological advancements means that as a business grows, so too can its forecasting capabilities. Cloud-based solutions allow entrepreneurs to adjust their forecasting models easily in response to changing circumstances, accommodating increased data volume or complexity as businesses scale. For instance, a startup that initially relied on a simple forecasting tool can transition to a sophisticated AI-driven model as its operations expand, ensuring that financial insights remain relevant and actionable.
Perhaps most importantly, technology fosters a culture of continuous improvement in financial forecasting. As machine learning algorithms learn from each new batch of data, the accuracy of forecasts increases over time. Entrepreneurs can incorporate feedback loops into their forecasting processes, utilizing past performance to refine future predictions. This iterative approach is invaluable in a business environment characterized by rapid change and uncertainty.
As we delve deeper into the intersection of AI and machine learning, it becomes clear that these technologies are not merely supplementary tools but rather essential components of a modern entrepreneur’s toolkit for financial forecasting. Understanding the underlying principles of AI forecasting and how machine learning functions will equip entrepreneurs to harness these capabilities effectively.
The next section will provide an introduction to the concepts of AI and machine learning, clarifying their applications in the realm of financial forecasting and emphasizing the strategic advantages they offer to tech-forward entrepreneurs.
Introduction to AI and Machine Learning
As the complexities of modern markets evolve, so too do the methodologies employed to analyze and predict financial performance. At the heart of this transformation are artificial intelligence (AI) and machine learning (ML), two interrelated concepts that have taken the realms of financial technology and forecasting by storm.
AI refers to computer systems designed to simulate human intelligence, possessing the capability to perform tasks such as learning, reasoning, and problem-solving. Machine learning, a subset of AI, specifically focuses on developing algorithms that enable systems to learn from vast datasets and improve their performance over time without being explicitly programmed for every task. This distinction is crucial for entrepreneurs eager to leverage these technologies; understanding how they work together opens up a world of strategic possibilities for financial forecasting.
In the context of financial forecasting, AI and machine learning algorithms can analyze enormous amounts of data at speeds and accuracies unthinkable for human analysts. For instance, while traditional forecasting models may use historical sales data as a foundation, AI-driven approaches can augment this data with real-time inputs such as external market conditions, social media sentiment, and emerging consumer trends. By processing this multifaceted information, AI forecasting can identify correlations and predict future financial scenarios with a degree of precision that improves decision-making.
One can draw a parallel between AI and seasoned financial analysts; however, AI’s ability to process and analyze data in real-time allows businesses to adapt swiftly to market fluctuations. Entrepreneurs can stay ahead of the curve, proactively adjusting their strategies based on insights gleaned from predictive models. This agility is vital for tech-forward entrepreneurs seeking to capitalize on fleeting market opportunities.
Moreover, as these technologies continue to evolve, the breadth of their applications within financial forecasting expands. Leveraging AI can revolutionize several aspects of forecasting, including but not limited to risk assessment, demand forecasting, and budget variance analysis. Each of these applications taps into unique data sources and analytical processes, but they all share the common goal of enabling entrepreneurs to make informed decisions grounded in empirical evidence rather than intuition.
As we explore the applications of AI in financial forecasting further, it is important to keep in mind that the effective incorporation of these technologies into business processes represents not only a competitive advantage but also a paradigm shift in how entrepreneurs can view their financial futures. The capabilities afforded by AI and machine learning empower innovators to anticipate challenges, seize opportunities, and create sustainable growth.
In the following section, we will delve deeper into specific applications of AI in financial forecasting, illustrating how these technologies can transform data into actionable insights and fundamentally enhance the entrepreneurial journey.
Applications of AI in Financial Forecasting
The incorporation of AI forecasting and machine learning into financial practices has ushered in a new era of accuracy and reliability in financial forecasting. One of the most pivotal applications is in demand forecasting, where businesses utilize AI algorithms to analyze historical sales data alongside real-time inputs. By considering factors like seasonal trends, economic indicators, and social media activity, these algorithms can predict product demand more precisely than traditional methods. For example, retail giants can anticipate sales fluctuations during holiday seasons or sales events, adjusting inventory and marketing strategies proactively.
Additionally, risk assessment stands out as another critical application. Financial institutions and startups alike leverage machine learning models to evaluate risks more comprehensively. By feeding models a variety of inputs—including credit scores, transaction history, and market trends—businesses can assess potential risks associated with loans or investments with greater accuracy. This capability not only enhances decision-making for lenders but also empowers borrowers with insights into their own financial stability.
Budget variance analysis is yet another area transformed by AI technologies. Rather than relying solely on static budgets prepared at the beginning of a financial period, entrepreneurs can utilize machine learning to produce dynamic budgets that adapt to evolving circumstances. Algorithms analyze variances between expected and actual performance continuously, offering insights into areas requiring corrective actions. With these advanced predictive capabilities, entrepreneurs can respond swiftly to discrepancies, ensuring that their financial health remains robust.
Moreover, AI forecasting empowers entrepreneurs by analyzing market sentiment. By scrutinizing vast amounts of data from various sources—such as news articles, blogs, and social media platforms—AI systems can detect shifts in consumer preferences or public perception that often precede market changes. This early awareness allows businesses to adjust their tactics swiftly, creating opportunities for competitive differentiation and innovation.
As these applications illustrate, the convergence of AI and machine learning within financial forecasting is not merely an enhancement of existing practices; it represents a transformational shift in how businesses operate strategically. Tech-savvy entrepreneurs who leverage these technologies unlock a treasure trove of data-driven insights, positioning themselves ahead of competitors who may still rely on more traditional, static forecasting methods.
Looking ahead, the next section will delve into the significant benefits that machine learning provides specifically for entrepreneurs, emphasizing how harnessing these advancements can lead to more informed, agile, and successful business decisions. Through a deep understanding of these benefits, tech-forward entrepreneurs can fully appreciate the value that AI-driven financial forecasting brings to their ventures.
Benefits of Machine Learning for Entrepreneurs
Machine learning offers a myriad of benefits for tech-forward entrepreneurs, transforming the landscape of financial forecasting and empowering businesses to operate with enhanced precision and agility. One of the most significant advantages is the ability to generate highly accurate predictions. By leveraging vast datasets and complex algorithms, AI forecasting minimizes human error associated with traditional forecasting methodologies. Businesses can expect forecasts that align closely with actual performance, enabling them to make more informed decisions about investments, resource allocation, and strategic planning.
Moreover, the speed of analysis is dramatically improved with machine learning models. Entrepreneurs can process and interpret large amounts of data in real-time, which was previously an arduous task requiring substantial time and manpower. This agility enables businesses to respond promptly to market trends, consumer demands, and economic shifts, allowing them to stay ahead of competitors who may rely on more conventional forecasting techniques.
Another remarkable benefit is the ability to uncover hidden patterns and insights. Machine learning algorithms can analyze data that traditional methods might overlook, providing a deeper understanding of market dynamics. For instance, a business might discover correlations between customer behavior and external factors, such as weather patterns or local events, that significantly affect sales. Armed with this knowledge, entrepreneurs can fine-tune their marketing strategies and product offerings to align with these insights, thereby driving growth.
Additionally, the integration of AI in financial forecasting promotes greater scalability. As startups or small businesses grow, their financial data becomes more complex and voluminous. Machine learning models are inherently adaptable, able to process increasing amounts of data without a decline in performance. This scalability ensures that entrepreneurs can maintain reliable forecasting capabilities, regardless of their business size or growth trajectory.
Consistency in forecasting is another vital benefit of AI technologies. Unlike human forecasters, whose predictions may vary widely due to individual biases or fluctuations in experience, machine learning models produce consistent outputs based on the underlying data. This reduces the level of uncertainty in financial planning, fostering a more stable operational environment.
Finally, utilizing AI forecasting enhances cost efficiency. By automating many aspects of data analysis and interpretation, businesses can decrease their reliance on specialized personnel for financial forecasting tasks. This not only reduces overhead costs but allows existing teams to focus on high-impact strategic initiatives that drive revenue and growth.
As the benefits continue to unfold, it is crucial to acknowledge that these advancements do not come without obstacles. Understanding the challenges and limitations of AI in forecasting is essential for entrepreneurs looking to maximize the potential of financial technology. The subsequent section will explore these challenges, providing insights on how to navigate them effectively in order to harness the full power of AI and machine learning in financial forecasting.
Challenges and Limitations of AI in Forecasting
Despite the transformative potential of AI forecasting and machine learning, entrepreneurs must navigate several challenges and limitations associated with these technologies. One major hurdle is the quality of data. Machine learning models heavily rely on vast amounts of accurate, high-quality data for training. If the input data contains inaccuracies, biases, or is insufficient in volume, the predictive power of the AI model will be severely compromised. For instance, a company attempting to forecast sales based on outdated or incorrect customer information may find itself with forecasts that deviate significantly from reality, resulting in misguided strategic decisions.
Another critical consideration is the complexity of models. While machine learning algorithms can analyze intricate patterns, their complexity can often make them less interpretable. This opaqueness poses a problem for entrepreneurs who need to understand the “why” behind predictions to communicate findings effectively to stakeholders or to make adjustments based on insights derived from the forecasts. Transparency is crucial in business, especially when decisions involving substantial investments are at stake.
Moreover, the cost of implementation can be daunting for smaller entrepreneurs or startups. Integrating AI forecasting into existing financial technology systems may require significant upfront investment in not only software and hardware but also training staff to utilize these advanced tools effectively. This can deter small business owners who may be hesitant to commit large budgets to technologies that have a steep learning curve and upfront costs.
In addition, entrepreneurs must remain vigilant about the ethical considerations surrounding AI and machine learning, particularly in terms of data privacy and security. The prevalence of data breaches and growing public concern regarding consumer privacy can complicate the data collection process required for effective AI forecasting. Businesses must adhere to regulatory frameworks and ethical guidelines, which can in turn impose limitations on data utility and flexibility.
Furthermore, reliance on AI forecasting can lead to a false sense of security. Entrepreneurs might underestimate the value of human intuition and experience in their decision-making processes. While AI can produce sophisticated models, many factors affecting market dynamics may be outside its scope, such as sudden economic shifts or unprecedented global events. Hence, an overdependence on machine-generated forecasts may result in missed opportunities or unforeseen threats.
As entrepreneurs confront these challenges, it’s essential to explore effective strategies for overcoming them. Techniques such as investing in quality data acquisition, ensuring model transparency through simpler interpretations, and balancing AI forecasts with human insight can go a long way in enhancing the effectiveness of AI-driven financial forecasting.
Recognizing and addressing these obstacles is crucial for those in the entrepreneurial realm to leverage the true potential of AI forecasting successfully. As we transition to the next section, we will delve into the future trends in financial forecasting with AI, illuminating the paths entrepreneurs can take to harness these advancements for sustainable growth.
Future Trends in Financial Forecasting with AI
As we stand on the brink of an exciting era in financial forecasting, AI and machine learning technologies are set to redefine not only how entrepreneurs approach predictions but also the very framework of decision-making in business. One significant trend is the increasing accessibility of AI tools. As the cost of computational power continues to decline and open-source platforms proliferate, even smaller enterprises are now able to harness robust AI forecasting capabilities that were once the purview of large corporations. This democratization of technology means that entrepreneurs can implement sophisticated forecasting models without exorbitant initial investments, leveling the playing field in various markets.
Another promising trend is the integration of real-time data into forecasting models. The rise of the Internet of Things (IoT) and advancements in data analytics allow businesses to continuously gather and analyze data from numerous sources. By leveraging real-time data, AI forecasting systems can adapt to changing market conditions swiftly, providing entrepreneurs with dynamic insights. For instance, retail businesses can track in-store customer behavior and purchasing patterns in real-time, allowing for more accurate inventory predictions and promotional strategies that align closely with consumer demand.
Moreover, we are witnessing an increased focus on collaborative AI, which integrates human intuition with machine learning capabilities. Forward-thinking entrepreneurs are recognizing the necessity of blending the analytical power of AI with the unique insights that human experts provide. Collaborative AI models can harness the strengths of both machines and humans, leading to improved forecasting accuracy and more nuanced strategic decision-making.
As AI technology evolves, we can also expect the rise of automated machine learning (AutoML) tools. These applications simplify the process of developing machine learning models, enabling entrepreneurs without extensive technical expertise to build effective forecasting solutions. AutoML platforms can analyze historical data, select the best algorithms, and fine-tune parameters to better fit specific business contexts. This shift not only accelerates adoption rates but also empowers entrepreneurs with actionable insights that would have otherwise remained out of reach.
Additionally, the implementation of explainable AI (XAI) is becoming increasingly important. As entrepreneurs increasingly utilize AI forecasting tools, understanding the rationale behind predictions will be critical. XAI initiatives are designed to increase transparency, allowing users to grasp how machine learning models arrive at their conclusions. This promotes trust in AI-driven forecasts and encourages entrepreneurs to act on these insights confidently.
Lastly, as digital innovation continues to unfold, sustainability and ethics are emerging as vital considerations in AI forecasting. Entrepreneurs are beginning to recognize the importance of developing ethical AI systems that not only comply with regulations but also contribute positively to societal progress. Forecasting models that take into account environmental, social, and governance (ESG) factors are being favored, leading to responsible business practices and long-term value creation.
As these trends unfold, entrepreneurs must prepare to adapt and integrate them into their financial strategies. Looking ahead, the future holds vast potential for those willing to embrace AI and machine learning in their forecasting processes. By remaining informed and adaptive, they can leverage the advancements in financial technology to drive sustainable growth and innovation in their enterprises.
In conclusion, as we summarize these future trends in the next section, it will be essential to highlight key takeaways that entrepreneurs can focus on to optimize their use of AI forecasting and ensure their businesses stay ahead in an increasingly dynamic economic landscape.
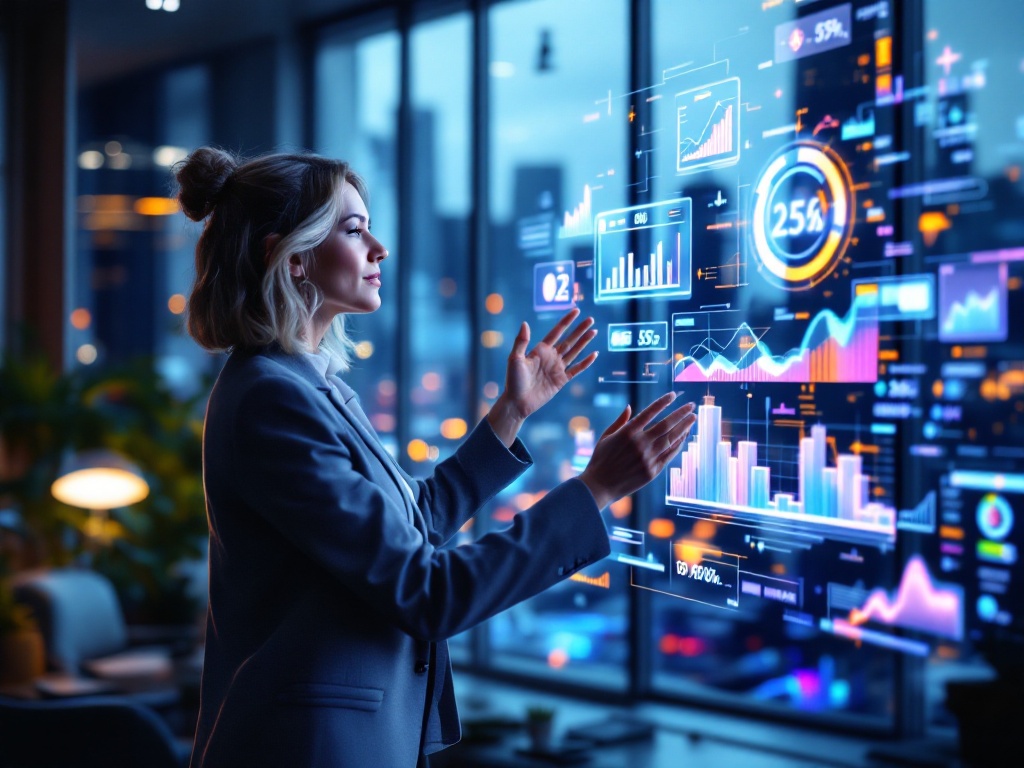
Conclusion and Key Takeaways
As we conclude our exploration of AI and machine learning in financial forecasting, it’s clear that entrepreneurs stand at the helm of a transformative wave within the financial technology landscape. The trends discussed earlier not only elucidate the potential benefits of integrating these powerful tools into forecasting practices but also underscore the urgency for entrepreneurs to remain agile and informed.
To effectively harness AI forecasting, it is vital for entrepreneurs to prioritize accessibility. By adopting affordable AI tools and open-source platforms, businesses of all sizes can utilize sophisticated forecasting models. This change fosters an environment where smaller enterprises can compete on a larger scale, making informed decisions based on advanced analytics rather than limited historical data.
Another key takeaway is the necessity of real-time data integration. Entrepreneurs who actively seek to incorporate IoT data and other real-time analytics into their forecasting models will gain a significant edge over competitors. The capacity to adjust strategies swiftly in response to dynamic market fluctuations is no longer a luxury; it is becoming a standard expectation for businesses aiming for success.
Collaboration between AI systems and human expertise should not be underestimated. As technology advances, the synergy between machine learning capabilities and human intuition is expected to yield improved predictive accuracy. Entrepreneurs should leverage this collaborative potential, encouraging an organizational culture where data-driven insights complement strategic thinking.
Moreover, embracing automated machine learning (AutoML) tools will empower even those without deep technical expertise to generate meaningful forecasts. As these tools simplify the model-building process, entrepreneurs can focus more on interpreting results and translating insights into actionable strategies rather than spending excessive time on the intricacies of model creation.
The implementation of explainable AI (XAI) must also be prioritized. Building trust in AI forecasting means ensuring transparency in how predictions are generated. By investing in accessible AI systems that elucidate their decision-making processes, entrepreneurs can encourage wider adoption of AI insights within their teams, leading to informed, confident decision-making.
Finally, the growing need to consider sustainability and ethical implications in AI forecasting cannot be overlooked. Entrepreneurs are increasingly recognizing that financial forecasting should align with responsible business practices. Incorporating environmental, social, and governance (ESG) factors into forecasting models not only mitigates risks but also promotes long-term value creation.
In summary, the evolution of financial forecasting through AI and machine learning is reshaping how entrepreneurs operate, transforming opportunities for growth and innovation. As these technologies continue to advance, so too must the strategies of those looking to stay competitive. Equipping themselves with knowledge, integrating real-time data, embracing collaboration, and prioritizing ethical practices will be essential for entrepreneurs seeking to make AI-driven financial forecasting a core aspect of their business strategy.
In the next section, we will delve deeper into practical steps entrepreneurs can take to implement these trends effectively, ensuring that they are not just passive observers of the evolving landscape but active participants driving their businesses toward success.
Follow us on our socials:
Facebook: https://www.facebook.com/MyBusinessGuysCom
Instagram: https://www.instagram.com/mybusinessguyscom/
LinkedIn: https://www.linkedin.com/company/my-business-guys/